A Tale of Two RCTS: The Value of Evidence in Ed Tech Research & Development
- Jeremy Roschelle
- Mar 17
- 4 min read
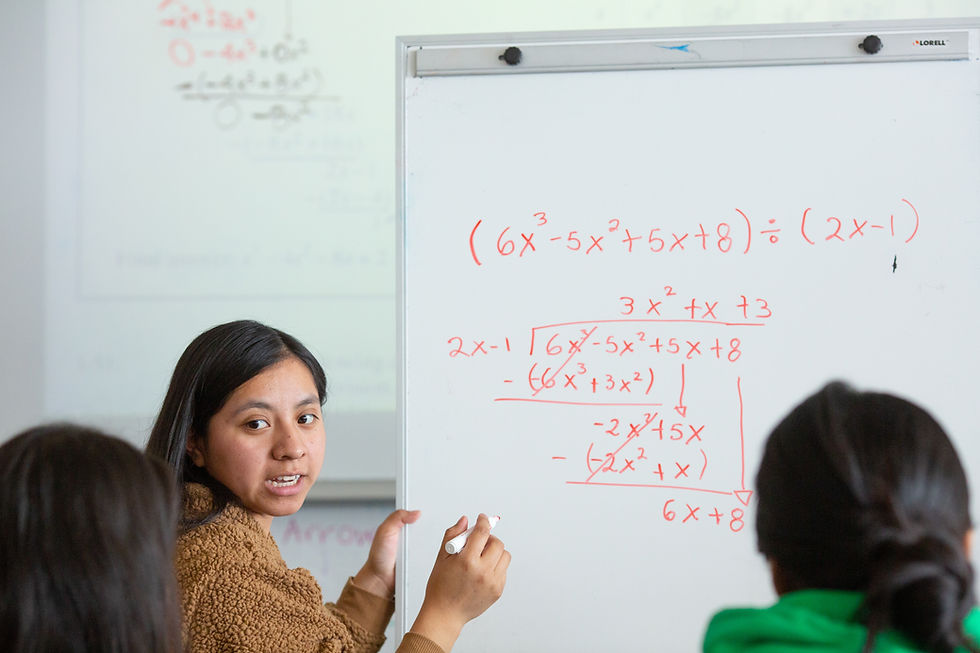
Between 2012 and 2014, I led the teams that conducted randomized controlled trials of two adaptive technologies for mathematics classrooms, ASSISTments and Reasoning Mind. The evidence showed that only one worked. I was surprised, as both came into our studies with strong prior evidence and state-of-the-art theoretical rationales. Now, more than a decade later, I better understand which insights from the research projects have stood the test of time. It turns out that "what works" isn't the whole story.
A “Randomized Controlled Trial” (RCT) is a type of research design that randomly assigns students to different groups, with at least one serving as the control group. This enables researchers to make an inference that one or the other alternative caused any observed impacts on student learning. Because of this power, RCTs are considered the "gold standard" for determining "what works."
Now let's consider what worked in the RCTs I led for ASSISTments and Reasoning Mind. It’s worth noting that the two platforms were not compared to each other but instead compared to unchanged classroom practice as it existed at the time.
ASSISTments is an online mathematics platform that provides support as students work on mathematics problems. In our implementation, students used the platform to enter their answers online as they did nightly mathematics homework, and when they did so, they received feedback on whether their answer was right or wrong. They also got hints and tutorials to help them improve and resubmit their answers right away. Teachers received an easy-to-read report that helped them see which homework problems were difficult for students and what the common wrong answers were, and they were coached to use this report to guide their in-classroom homework review.
Reasoning Mind was an online mathematics curriculum that used an Artificial Intelligence (AI) algorithm to diagnose math problem-solving issues in the classroom and provide the student with specific experiences to address their individual needs. Teachers received reports that enabled them to spot which students needed personalized attention and on what mathematics concepts and skills. Students spent a lot of time working alone at computers, so teachers spent less time managing the whole classroom and more time working with individual students.
Which platform do you guess had a bigger impact on student achievement than existing instructional practices?
In our research, ASSISTments proved to have a meaningful positive impact on student learning. Reasoning Mind was statistically indistinguishable from existing instruction. Today, ASSISTments has millions of users; Reasoning Mind no longer exists.
From the ASSISTments study, the enduring lesson I learned was about the power of feedback and how to get it right. The artfulness of ASSISTments is not just that it gives feedback but that the feedback is delivered in a way that leads both students and teachers to change their behavior. For students, they are not just passively receiving feedback but using it to revise their answers in real time, in part by learning from associated hints and tutorials. For teachers, the platform changed how they reviewed homework during class. We found that successful ASSISTments teachers discussed fewer problems with their students but with greater depth. We also learned that feedback works when integrated into a weekly routine that takes between 30 to 60 minutes, and that it can be particularly beneficial to struggling students
From the Reasoning Mind study, we learned to think more clearly about why AI or personalization might not always be beneficial. Here are some thoughts we had as the study ended:
The classrooms that used Reasoning Mind were less social and collaborative, even though the students and teachers in the experiment stated that they preferred to learn math socially (and other research establishes that social learning of mathematics can be powerful).
In some classrooms, Reasoning Mind assigned students to an unusually wide range of mathematics tasks, which might have made it harder for teachers to know what students are working on, and thus harder for teachers to help students.
It was a big effort for teachers to change their routines to use Reasoning Mind as intended; it took a lot of support for teachers to enact the new classroom model. As we watched the teachers in their new, unfamiliar roles, we saw some issues with instructional quality.
So what's the value of evidence? Knowing "what works" is important because that can drive our quest to understand the big ideas that influence student outcomes, such as the power of feedback. But these large, rigorous studies also make people think about the conditions necessary for strong student learning and what might be getting in the way.
A decade later, educators can choose among ASSISTments and many other math products that deliver feedback as students solve problems. Here is one classic research article on feedback. No matter the platform, I'd want it to feature adaptivity and feedback, and also prompt important behavioral changes that align with research insights about excellent mathematics teaching.
Educators can choose among many AI-based products that adapt to students' strengths and needs. I'd want them to ask questions like: Are these making it easier or harder for teachers to work meaningfully with their students? Are they strengthening or blocking other valuable classroom practices, like social and collaborative learning? How hard is it for teachers and students to use these products as recommended, and as necessary to deliver their intended benefits to student learning?
The value of evidence is learning what big ideas drive outcomes, sharpening our thinking about how students and teachers need to change to benefit from these technologies, and making educators aware of what could go wrong when adopting a new classroom technology. The value of evidence is also in making us think hard about what "good implementation" looks like, because an infrequently-used or poorly-implemented educational technology has no chance of achieving its desired contributions to improved learning.
Jeremy Roschelle is the Executive Director of Learning Sciences Research at Digital Promise.